
Your path to AI
When it comes to embracing new technologies like Artificial Intelligence (AI), it’s easy for companies who are curious to be influenced by extreme examples of success or failure. Indeed, a lot of the stories of failure and disappointment are often based on exaggerated expectations.
However, such stories are not necessarily helpful or instructive for a company that is considering AI for its business. Instead, a more realistic analysis of the challenges and opportunities at play is in order. In this white paper, we will analyze both sides of the AI issue and help you discover the right way to approach your AI journey.
Consider Key Challenges
Let’s start with the AI opportunity. McKinsey estimates that AI techniques have the potential to create up to $5.8 trillion in value annually across nine business functions in 19 industries.1 Despite this tremendous potential, it’s worth remembering that AI is still in its early days, and therefore, as with any new technology, there are some initial challenges that will need to be tackled.
Gartner predicts that just 5% of organizations will gain value from AI through 2019 because most teams lack the talent needed to manage AI projects.2 Lack of skill, which can also include the organization’s culture, is one of the key factors that can cause an AI project to fail.3 This underscores the importance of choosing the right development partner that can provide the necessary skills and facilitate knowledge transfer and exposure to industry best practices in order to build up your in-house capabilities.
Another reason that AI projects fail: the lack of integration between the AI project and existing processes and systems.4 To be successful, an AI project cannot be an isolated pilot; its integration and eventual expansion must be envisioned and planned from the start, and it should involve several functions and leverage different types of expertise across the company.
What are the top challenges with cognitive technology?

Based on our experience, there is another item that should be on the list of key challenges: data.
The availability of the data you need, as well as the quality of the data being used, can be significant factors in an AI project’s success or its failure. Often, companies may not recognize this is a problem until after they have already started their AI project.5 At this point, poor quality data or not enough of the right data, can completely derail your project.

So, what are the ingredients for a successful AI project? The very nature of AI projects—the data used, the other technologies in play, the strategy employed—means that each one will be different. However, in our experience, there are some common themes that the most successful AI projects share.
What successful AI projects have in common
- Identify the problem and get clear on the value: Don’t get lost in “cool” technology. Have a clear vision of the problem and the value it needs to deliver for your organization. The value should be understood in both quantitative and qualitative terms.
- Rethink business processes: Don’t just think that incremental improvements are the answer when a transformation could be in order. While cognitive capabilities could improve some operations or enable and augment several activities and services, subsequently it might be necessary to revise, re-design or even eliminate related processes.
- Be user-driven: The AI age relies on business insight to drive value. Real business users should be on the project team to understand the problem, to brainstorm for potential solutions and for testing to ensure that the solution is providing value.
- Involve experts from the domain where the project will be deployed: As we move from a programmatic and rules-based era to a probabilistic one, training is key and it must be continual. This will require periodic reviews by subject matter experts to train the model and ensure that their knowledge is infused into your AI solution. This validation will allow you to have greater confidence in the system and its ability to automate decision making.
- Start small to learn: We have realized the best results when organizations start small, create proof points and expand quickly. With AI, it’s important to remember that the journey and the destination are both important.
- Live your solution, learn and iterate: Your AI solution should continue to grow and be iterated upon, especially since you already have the team, the knowledge base and the infrastructure in place.
Finally, remember that a little scepticism can be a good thing! If it sounds too good to be true, it probably is. Instead, it’s important to set realistic expectations for any AI project.
Companies are reaping the benefits AI
Intesa Sanpaolo
Cogito has allowed us to quickly achieve the Group’s digital strategy objectives for innovation, helping us improve the quality and experience of our online services. We wanted a new and intelligent tool capable of bringing our customers closer to the bank, and we are very pleased with the results.
Francesca Nieddu, Director of Customer Experience and CRM, Intesa Sanpaolo, and Massimo Tessitore, Head of Integrated Multi channels, Intesa Sanpaolo
A leading Italian banking group.
The challenge
Find an innovative and qualitative way to enhance the digital experience of customers
Solutions
Cogito®, Expert System’s cognitive platform, was chosen to enhance its customers’ digital experience. A combination of virtual agent and intelligent search system, the solution based on Cogito analyzes the meaning of users’ words and phrases to understand their intentions with maximum precision and Cogito responds with the requested information or proposes relevant actions such as direct access to wire transfers, deposits, bill payment or any of the numerous other home banking options. It also helps fill in forms with information such as amounts or recipients of transactions.
Benefits
- Users no longer have to worry about selecting the “right” search expressions because they can be understood using everyday phrases.
- Compared to other cognitive computing solutions that typically involve long implementation cycles, Cogito is both quick to implement and scalable, resulting in a rapid ROI.
- Only six months after its launch, the service is achieving a fast adoption rate with 30% of customers already using the service regularly.
Dow Jones
A trusted source of business news.
The challenge
Packaging news stories and insights from a database of more than 1.3 billion articles in 28 languages so that this information becomes actionable for customers and doing it efficiently and at scale, is far from easy. Dow Jones decided to leverage AI to process unstructured content and deliver a seamless discoverability experience to its clients.
Solutions
At the heart of the Dow Jones information powerhouse is an AI-driven pipeline that processes 1.5 million news articles every day, extracts their details and creates the Dow Jones Identifier metadata and knowledge that drive Dow Jones’ products.
Benefits
- At Dow Jones, AI helped turn a billion articles into insights. Clients benefit from a more effective discovery experience that pinpoints the events, people and companies that count on their research.
ENI
An important integrated energy company.
The challenge
To optimize information for the activities of information management and marketing.
ENI chose Cogito for search and analysis of strategic information to improve the internal management of knowledge assets and information retrieval and for advanced research to monitor external sources of information.
Benefits
- Reduced time and costs for information management using data mining, semantic search, content navigation and automatic categorization.
- Monitoring of markets and competitors for competitive advantage.
- Advanced collaboration platform for knowledge workers and analysts to share intellectual capital.
Lloyd’s
Artificial Intelligence drives productivity by changing the way we benefit from data, and Lloyd’s continues its history of innovation. Expert System’s cognitive applications help us meet our strategic objective and evolve business models through new applications.
Craig Civil, Head of Data Innovation, Lloyd’s
Zurich Insurance Group
At Zurich, our focus is on the modernization, simplification and digitalization of our business and ways of working. Investing in state-of-the-art technology and automation, and making better use of our data are important steps towards a digital mindset. The cognitive computing solutions of Expert System enhance our efficiency and effectiveness and thus help us to improve customer services and propositions.
Giovanni Giuliani, Group Chief Strategy, Innovation and Business Development Officer, Zurich
Crédit Agricole
Expert System really boosted the project. The first pilot was so easy to implement that we were soon presenting the features to our users and showing them the added value of the Cogito solution. The Media Topic module, mapping global media trends in real-time, made a particularly powerful instant impression. Cogito’s strength lies in its implementation speed, its ease of use, and the coverage of its out-of-the-box knowledge graph and classification plan. The tools’ flexibility allows us to constantly evolve features based on our lead users’ feedback, which they find thrilling.
Gautier Sartorius, Securitisation Chief Information Officer, Global Markets Division at Crédit Agricole CIB
CIB is the Corporate and Investment Banking arm of the Crédit Agricole Group, the world’s 13th largest bank.
The challenge
Automating the analysis and processing of public information and the management of unstructured data. Its web portal, PanOptes, uses collective intelligence to provide users with the most relevant information based on semantic analysis of public data flows.
Solutions
PanOptes uses Expert System’s Cogito to semantically process large volumes of text content from various sources. Based on artificial intelligence algorithms that automate unstructured data processing, Cogito is an integral part of the PanOptes platform. The solution combines a semantic search engine with a virtual agent capable of accurately understanding the content coming from the data sources, categorizing it, identifying opinions and grouping it by topic or geographical location.
Expert AI: From Chaos to Order
Expert System recently deployed its Cogito text analytics application at a large European insurance company. The process reduced the time to review a claim from one hour to a few seconds, saving some 40,000 hours of manual work annually and improving accuracy. The ROI on the technology investment? $5 million per year. Forrester, 2018.6
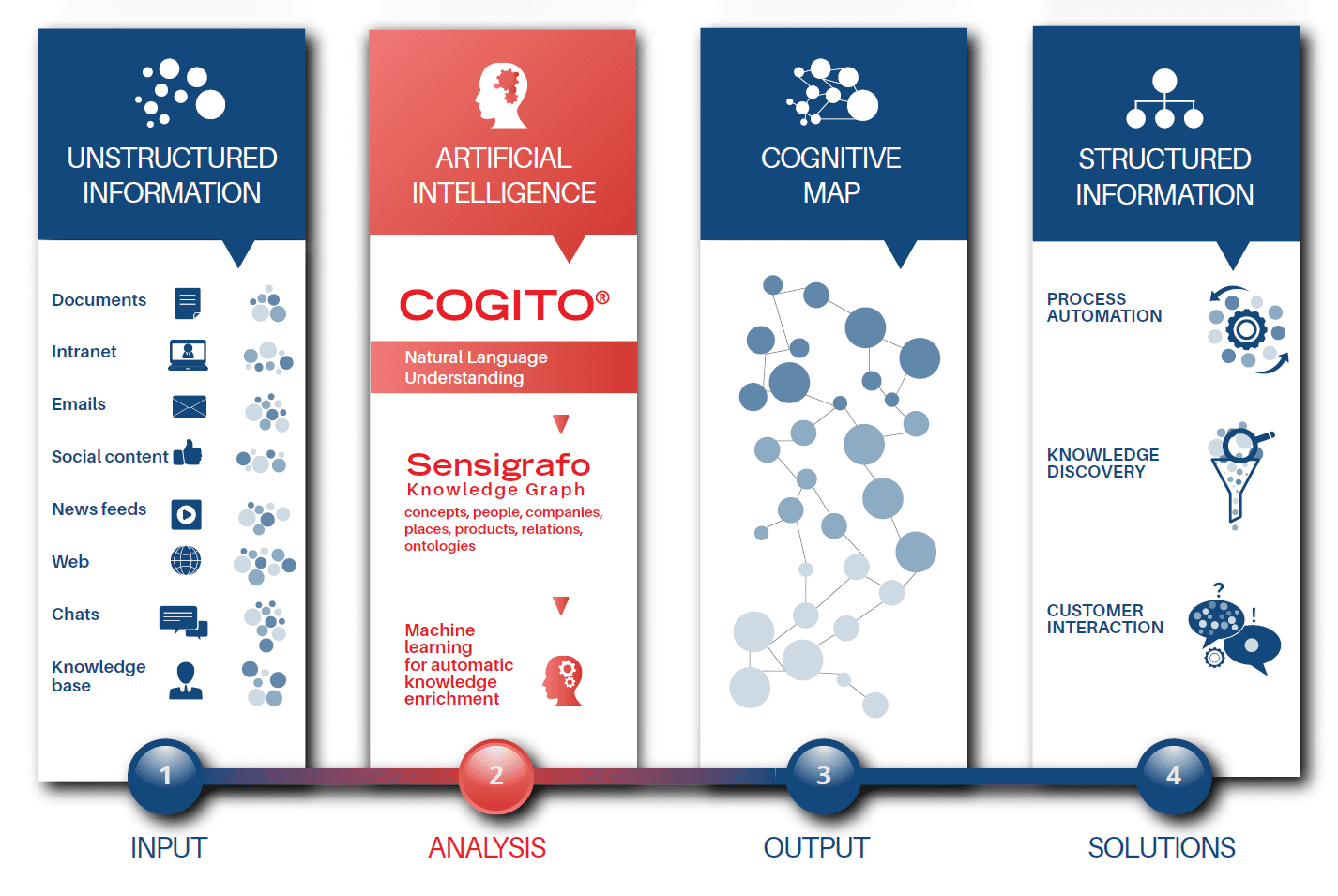
Start your AI journey with us: Artificial Intelligence in action!
With 300+ AI projects and counting, Expert System has consolidated a winning AI methodology in four steps. What makes Expert System unique compared to other vendors is that our technology is not a black box: With our demo, you’ll be able to immediately test how it works.
Phase 1: Engagement
In this phase, it’s important to define the scope of engagement. The priority for us is to assess your context and to better understand your strategy, knowledge and aspirations for AI. In the meantime, we’ll share our background, experiences and technology through use cases and through a demo of the Cogito platform.
The demo highlights a sampling of the features that make the Cogito AI technology truly unique, such as the knowledge graph and the semantic engine.
We’ll be able to demonstrate a range of text analysis features, from disambiguation and grammar analysis, to identification of categories, entities and relations between entities, the most important elements in text and sentiment monitoring. The demo is a real “hands on” view of the value of AI, and it will show the potential of Cogito and how it can be applied to your use case.
Phase 2: Problem definition
We work with you to define the scope of the project, highlight the issues, understand the expected results (qualitative and quantitative) and identify the key indicators to measure them.
Good problems to start with have several criteria:
- It’s an area that is important for your business
- The data necessary for addressing the problem is readily available (otherwise the bulk of your time will be spent here)
- You can work with the data (or a relevant subset of it) comfortably on a single machine
A core element of this phase will be analyzing the business case and defining its critical components, which will be verified through the proof of concept.
This will also give us the opportunity to verify and test any questions that arise as requirements are defined.
Phase 3: Proof of concept
Implementing an AI-based solution requires expertise, time and analysis. Not all use cases that appear to be suitable for AI are, or are in a way that has a positive cost/benefit ratio.
To understand whether an AI-based project can be successful, the best way is almost always through implementing a proof of concept (PoC). This is especially relevant for text analytics, where Gartner recommends conducting “flash or quick proofs of concept to test a vendor’s capability with a small sample of data to narrow down the options, then plan for a full ontology of domain-specific data for a comprehensive evaluation of the tools’ capabilities”.7
For emerging technologies such as A I, Pa oC is generally the most cost-effective path to show quick wins and build confidence.
A PoC is a “closed” but working solution that can be evaluated and tested subject to clear criteria, from understanding requirements to delivering success. Based on the PoC, the next step will be creation of a detailed approach and a plan for implementation, including cost estimates, timelines and a high-level work estimate of what will be required to take the PoC to the next step and begin building the real project.
For any AI project or program, PoCs enable decision-makers to:
- Deliver more immediate value
- Gain skills and experience
- Test the technologies being used
- Identify and resolve potential data issues
- Highlight potential impacts on the project context (infrastructure and processes)
- Gain confidence in AI
Phase 4: Scaling up to the project
The problem has been defined, the solution designed, the data profiled and modelled. The PoC has been built, tested and deployed. So, what’s next? You can do a number of things to ensure that your PoC remains a success story so that you can build upon it in support of a broader AI strategy. You can go on to:
Tune and optimize the PoC solution. As time goes on, you will develop more skills for improving and enhancing the AI solution you have deployed. You can optimize software around areas such as data curation and labelling and experiment with, train and deploy new models that may provide even better results.
Scale-out to other business scenarios. Your PoC may be applicable for other parts of your business. You can adopt a portfolio approach to manage how you extend the PoC across a wider user base.
Our approach in a snapshot
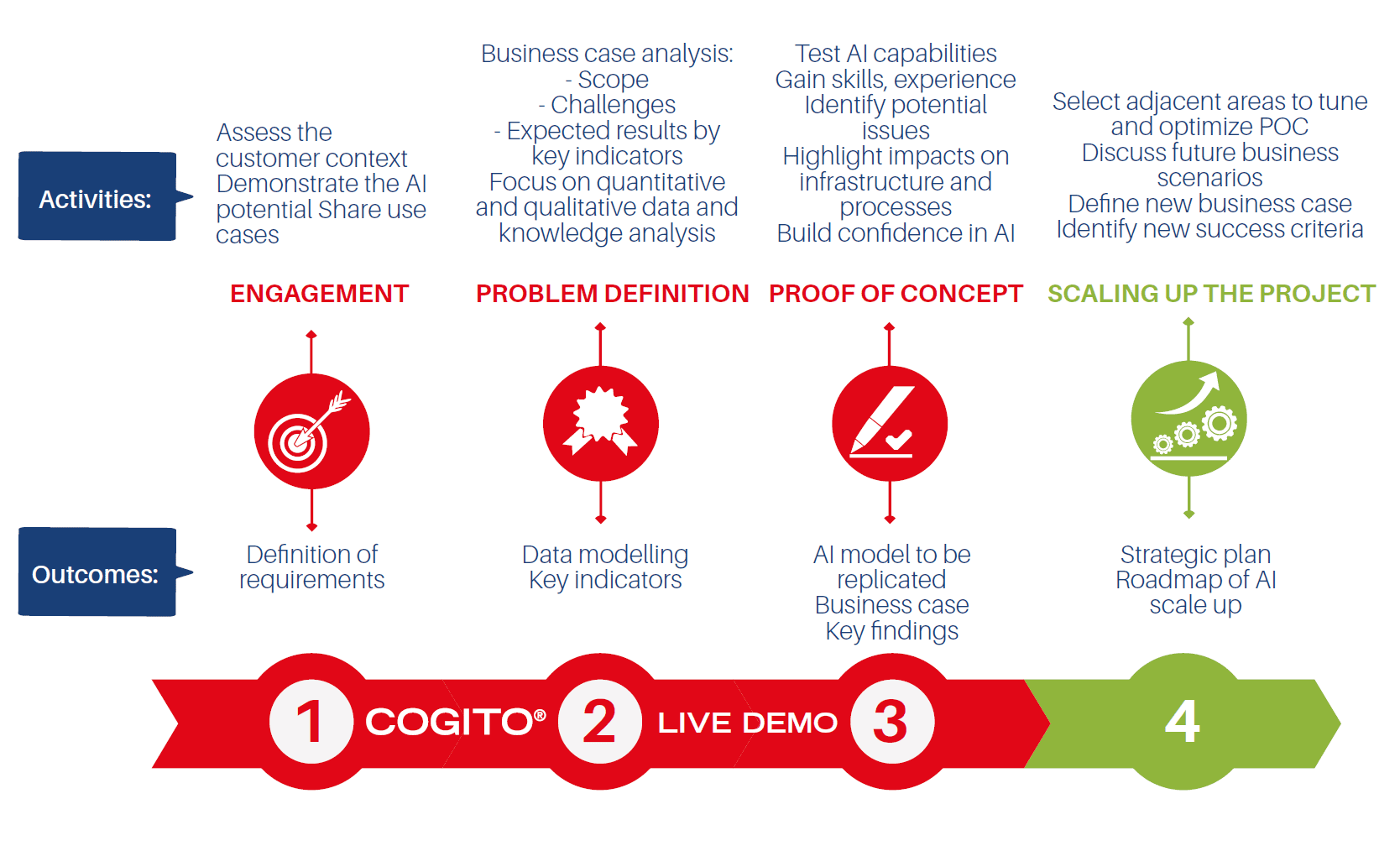
A PoC is the best approach to AI. A PoC provides all of the key elements necessary to determine if and how to proceed with an Artificial Intelligence project in a short timeframe. Generally, the first phase may require 6 to 10 months, depending on the selected area, the complexity and the type/ number of key indicators that are needed to monitor and verify.
It’s no secret that AI and unstructured data projects are risky. Success is far from guaranteed. Mitigating the risk requires a knowledgeable partner that can support your organization with the necessary resources and expertise.
End Notes
- https://www.mckinsey.com/featured-insights/artificial-intelligence/notes-from-the-ai-frontier-applications-and-value-of-deep-learning
- https://www.gartner.com/doc/3714236/cool-vendors-ai-core-technologies
- https://www.gartner.com/smarterwithgartner/how-to-build-a-business-case-for-artificial-intelligence/
- https://www2.deloitte.com/content/dam/Deloitte/us/Documents/deloitte-analytics/us-deloitte-cognitivesurvey.pdf
- https://www.cio.com/article/3254693/artificial-intelligence/ais-biggest-risk-factor-data-gone-wrong.html
- https://www.forrester.com/report/Look+To+Four+Use+Case+Categories+To+Push+RPA+And+AI+Convergence/-/E-RES141542
- https://www.techrepublic.com/article/gartner-here-are-4-critical-lessons-weve-learned-from-early-ai-projects/
- https://www.gartner.com/doc/3892564/market-guide-text-analytics